“How can we benefit from AI to make better pricing decisions?”
To many executives, putting AI to work in their business seems daunting. While AI is talked about a lot, actually implementing AI in an operational enterprise setting remains too much of a challenge to the majority. However, as AI technologies and talent become more accessible, the tide is starting to turn. More and more businesses are thinking seriously about where to deploy their first AI capability.
And that’s good news – because businesses that have traditionally had many Operations Research (OR) problems at their core, can now benefit from applying AI techniques to these problems. The aviation, travel and transport space are full of classic OR problems - yield management being a crucial one. Revenue managers in aviation can use AI to make better pricing decisions that drive profits - often in ways that perform better than the traditional OR techniques.
Recently, I was asked, “How can we benefit from AI to make better pricing decisions?” – a great question. Why? Because it shifts the conversation from theory to practice; from talking to doing.
For those with products (often time-bound) with dynamic prices, thinking about the advantages AI might give you, here are the 7 things to consider as you get started.
The 7 steps to becoming AI-ready for pricing
1. Test first to validate the opportunity
Do we know how much market elasticity there is to exploit? How much do we know already about how different prices affect demand? If no one is willing to buy at a higher price, we can’t put prices up any more anyway - so we may not need AI. Some of our customers weren’t doing any significant price exploration before - they already thought they were using the best prices. So, before we deployed a sophisticated AI pricing agent, we delivered some simple A/B pricing tests to confirm there was further elasticity in the market to exploit. This gave the business (and us!) enough conviction that this was an opportunity worth investing further in.
2. Instrument yourself
In order to understand market elasticity, and give any AI solution (likely a reinforcement learning agent) enough data to get started with, it’s much better if we can understand how customers have responded to different prices shown in the past. Most businesses will always capture the price they sold something at - even if it’s a dynamic price (be it an aeroplane ticket, a parking space, a taxi fare, etc). But crucially, many businesses don’t capture the times they offered something at a price and it WASN’T purchased. This information is crucial and really valuable to learn from - and it’s often not captured. If you don’t capture this data already, instrument your business to capture this data today, and we’ll speak again in a few months to analyse this data and understand the market elasticity better.
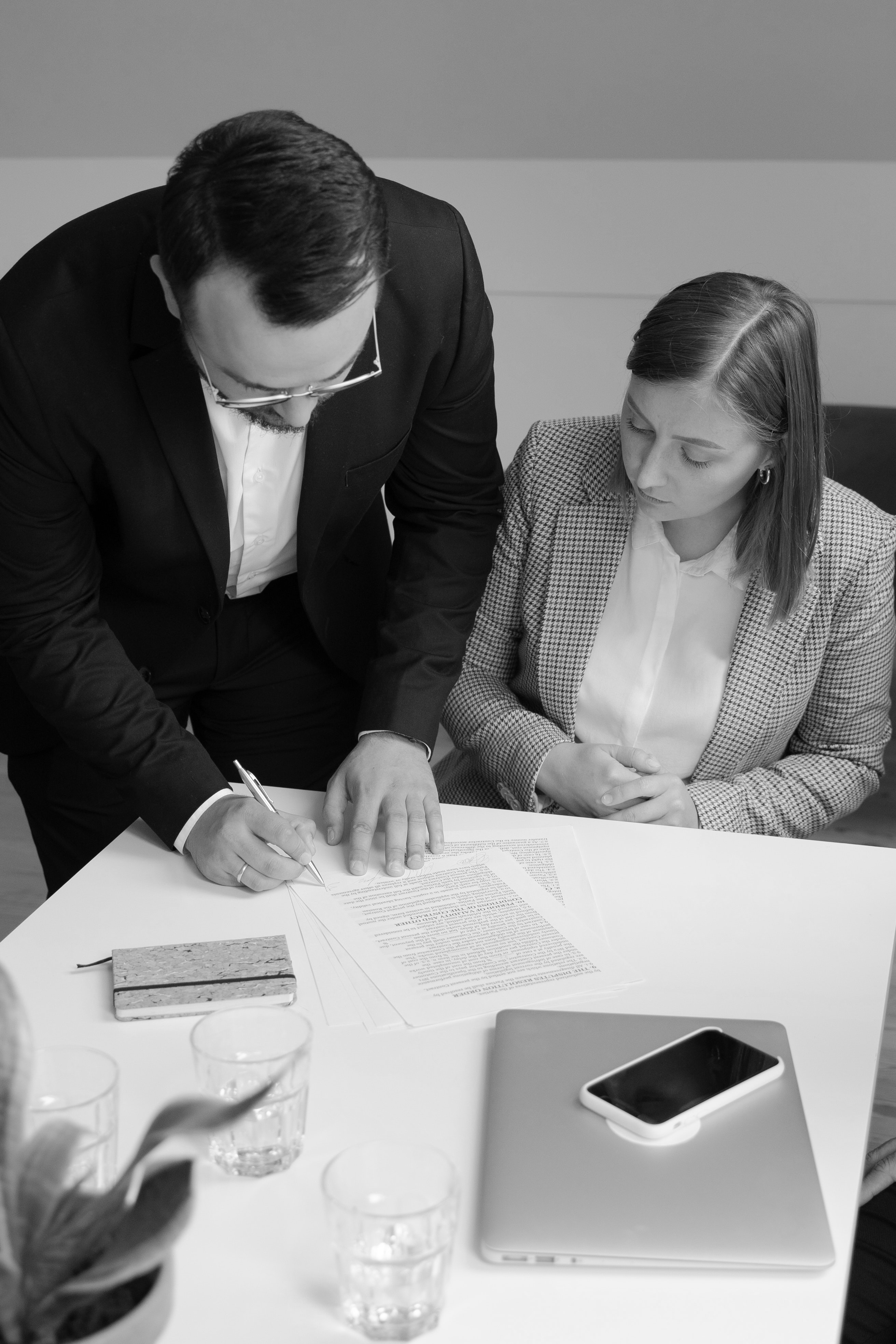
3. Define your objective function
Any AI needs to be absolutely clear on what it’s trying to achieve, which means you need to be absolutely clear on what your goal is. In a car parking example, an optimal price to maximise occupancy will likely be different from one which optimises revenue per space per day, or maximises total average booking revenue. Understanding the trade-offs of different objective functions and getting clarity and agreement on the goals of the pricing engine will set you up for success.
4. Think about disadvantaged customer segments you don’t want to exploit
If you ask an AI agent to optimise a price to maximise an objective function (maybe revenue, maybe profit, maybe a combination of profit and customer service metrics like wait time) - it will do it. But in reality, you may not always want the AI pricing agent to fully exploit and maximise the objective function you’ve given it. For example, as a brand, you may not want to be seen to be too exploitative in some instances (e.g. Uber surge pricing when there’s a natural disaster or a terrorist event). There may be some disadvantaged customer segments you don’t want to exploit. It’s important to acknowledge and articulate these guardrails so the AI agent knows its limits, and you have the ability to step in and course correct as and when necessary.
5. Be prepared to take risks
You don’t know something until you’ve tried it. Just because you’ve always charged £10 doesn’t mean you might be able to charge £12. Macroeconomic changes (the current cost of living crisis is a case in point) mean you continually need to experiment and test new prices in the market - and many of those experiments won’t give as good results. Getting the balance of exploration vs. exploitation right is a crucial aspect of implementing a reinforcement learning agent (and giving it guardrails on how far it can explore). Your business needs to be comfortable taking some risks (sometimes pricing things too low and making a loss, sometimes pricing too high and scaring away demand) to explore different prices in order to be able to benefit from exploiting the findings.
6. Set your communication plan
Depending on how much dynamic pricing is being done already in the business when you start to deploy it, how are you going to communicate it to your staff and your customers? What if a customer has always paid £10 and now they’re asked to pay £12? If they call up your call centre to query it, how do your call centre colleagues explain this? This is an important change management exercise to plan and get right before deploying.
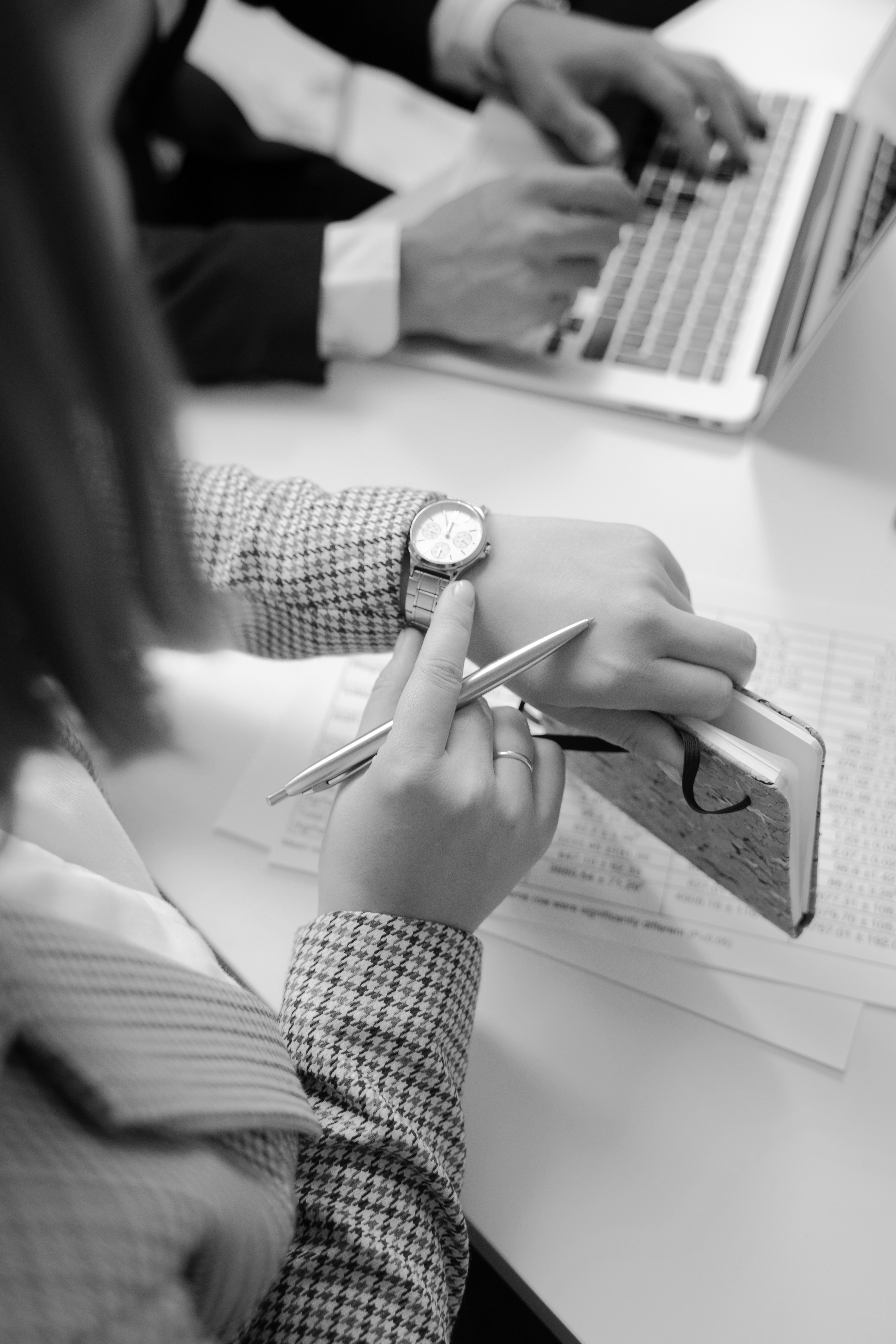
7. Prepare for your commercial team to evolve
Today, you might have a Revenue Manager in the business whose responsibility is to determine and upload new prices and tariffs each day. Just because you now have a reinforcement learning agent doing this more frequently, using more data to determine optimal prices more intelligently doesn’t mean the Revenue Manager is out of a job - far from it.
Their responsibility is still crucial, and uses their key skills that the AI can’t do: their role pivots to governing the RL/AI agent setting the prices - guiding it (telling it how far to explore and exploit - tweaking the guardrails), if necessary overriding and course correcting it (e.g. in response to knowledge the RL agent doesn’t yet have - a new bank holiday that’s just been announced, or a volcano that’s just gone off and grounded all flights).
Defining this new role for your revenue management team, and giving them the necessary support in training and tooling to help them in this transition is another important business process change management exercise to plan and get right before deploying.
Same question, new answer
Investing in AI is a long-term play as well as a short-term win. It’s easy to over-index on technology when starting a new AI project. So, it’s worth taking a moment to expand on the need to have the right people and processes in place.
There’s an old Einstein fable that explains why.
Einstein gave an exam paper to his class that was identical to one he had given a year before. His teaching assistant, upon noticing this, let him know.
“Professor, I’m not sure if you know, but this test is identical to the one you gave out last year.”
After a short pause for thought, he replied with something interesting.
“The test is the same – but the answers are different.”
The world, your business and the way your customers respond to prices are always changing. The environment that the optimiser operates in is in continual flux (which is why a continually learning reinforcement learning agent is a good approach).
But executives must also focus on the changing needs and roles of their people and processes as well as technology when it comes to operationalising AI. It often comes in the form of a well-prepped in-house team or trusted, long-term partner; a team around for the long haul.